For the past few months securing resources from COVID-19 has become a tough row to hoe for the ecosystem. With the sky-high technological demands to detect symptomatic individuals, elevated body temperature measurement is a key topic in the current pandemic situation. Thermal Camera- is one such solution which is widely being used to measure fever-like symptoms. Many OEMs have launched their solutions however evidence suggests that the ACCURACY of the cameras in temperature measurement is still an area of grave concern. To provide our society with a more reliable Thermal Camera Solution, OEMs need an AI-enabled camera design with a strong design architecture refined with accurate calibration. Let’s understand how these technologies work in the concept of Thermal Camera.
Thermal Camera Principle
Unlike CMOS/CCD cameras, thermal cameras have a microbolometer that is operated in infrared regions to capture the IR intensity of each of its pixels. The key to thermal imaging is that a hot surface emits more IR than the cold surface. A special optics focus all the heat radiations of a scene to its sensor array. All the pixel values can be then converted to respective temperature values, and the same can be represented as respective color codes (e.g, cold surfaces can be represented with blue color and hot surfaces with red color).
Calibration for High Accuracy
During this pandemic situation, the deployment of thermal cameras at various places for the measurement of body temperature demands high accuracy that can be maintained with the periodic calibration of the cameras. Having the shortest normal body temperature range (36.5C to 37.5C) with respect to the human body temperature range (35C to 40C) indicates the need for accurate measurement which can be fulfilled up to a certain level with the help of calibration. However, if we go without calibration that will lead to uncertain results with floating accuracy and thermal imaging cameras can provide low accuracy temperature values & relative temperature changes on the surface.
- A calibrator device sits in the thermal camera FoV with a constant temperature as a reference to the estimate of all other pixel temperature.
- Accuracy up to 0.3C can be achieved by using an external calibrator.
- Flat plate IR & Black body calibrators are commonly available calibration devices.
Other Parameters that Impact Thermal Camera Accuracy are:
Emissivity – It is the measure of object ability to emits IR energy
Atmospheric temperature – Cold, Wind, Heat, etc, all play an important role
Reflected Temperature – Thermal radiations come from other sources/objects that reflect the target we are measuring
How AI, Deep Learning and Thermal Camera work together?
The onset of the pandemic has accelerated AI-infused technologies to mitigate the spread of the virus. Thermal cameras have to be repurposed to enable them to accurately identify elevated body temperature. AI and Deep Learning technologies are our best bet. Thermal Cameras, when enabled with AI, helps its accuracy by
- Boosting hardware claimed accuracy with AI analytics
- AI-Based precise targeting of the region of interest which is the forehead of the person
The combination of running AI on a CMOS camera with a thermal imaging camera enables the automated temperature screening by detecting a person’s forehead. This helps in avoiding many false triggering events in normal thermal cameras, e.g A person holding a hot cup of coffee will trigger false measurement without AI. Image processing, analyzing techniques, and AI & Deep Learning algorithms are often used to negate false triggering.
Enabling Face Detection and Forehead Sensing in Thermal Camera
Face and facial landmark detection are done through MTCNN (Multi-Task Cascaded Convolutional Neural Network) which is an AI/ML-based 3 stage algorithm to detect the bounding boxes of faces in an image along with their 5 point face landmarks. Each stage gradually improves the detection results by giving its inputs through a CNN, which returns bounding boxes with their scores, followed by non-max suppression.
Stage 1: The input image is scaled down multiple times to build an image pyramid and each scaled version of the image is passed through its CNN.
Stage 2 & 3: Image patches get extracted for each bounding box and resize them (24×24 in stage 2 and 48×48 in stage 3), forward them through the CNN of that stage. Besides bounding boxes and scores, stage 3 additionally computes 5 face landmarks points for each bounding box.
The output from MTCNN will be a bounding box for the face with facial landmarks marked on it.
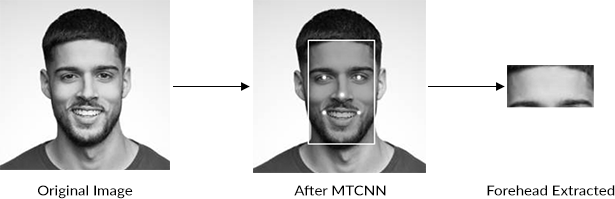
Since the coordinates of the bounding box and both the eyes are available forehead extraction can be easily done by cropping.
Estimated ROI can be co-related with thermal camera FoV when both CMOS and thermal cameras are placed side by side to calculate the temperature readings. A combination of averaging and peak finding algorithms shall be used to determine the optimum temperature measurement of ROI.
How does VVDN help OEMs?
VVDN’s Vision team comes with proven and deep expertise in Camera Design, Calibration, Computer Vision, AI on Edge, Deep learning techniques, Product Validation, and System Integration. VVDN addresses the OEMs concern for a fully reliable thermal camera with its extensive engineering and manufacturing capabilities. VVDN with its reusable frameworks and components can help OEMs with effective and accurate thermal camera solutions powered with AI and Deep Learning in a FASTER TIME TO MARKET.
For any such requirement feel free to contact: info@vvdntech.com
Click here to read more about VVDN’s camera expertise.